Co-authored by Gokul Kumaravelu.
Cover image produced with help of Stable Diffusion on Hugging Face
"Any sufficiently advanced technology is indistinguishable from magic." - Arthur C Clarke
From the first powered flight to the first smartphone, technology has given man superpowers that inspire fear, wonder, and fascination. AI is the latest addition to that list of ‘magical’ inventions.
The world changes every day but in the days that followed the release of ChatGPT, DALL-E, and other generative AI, people collectively felt a foundational shift towards something that pushed our imagination. Prompt-based generative AI has ushered in a new era where AI acting as humanity's magic co-pilot will help us create almost anything we imagine.
Generative AI models are just the latest child in a long line of our attempts to fulfil the promise of AI that started with Herbert Simon and Allen Newell predicting in 1957 that a computer would beat a human at chess.
In less than seven decades of John McCarthy coining the term “Artificial Intelligence,” AI is not just beating humans at chess, it is also helping nuclear scientists tame plasma hotter than the center of the sun.
And we are just getting started. By the time you read this, some of our predictions might already be realized with research breakthroughs and developments happening almost every week. For example, within the first week of writing this draft, Meta released a multi-modal generative AI that produces both image and text outputs from one prompt better than DALL-E 2. DALL-E’s API became publicly available on November 3rd, 2022.
AI researchers, founders, and governments are building models, apps, and collectives to push us closer to a future where you can think of any idea and instantly bring it to life. A one-person unicorn built by one brilliant mind and a team of AI might be a very real possibility in the next decade.
Over this multi-part series, we will outline our thesis for the space to inspire and inform not just today’s founders but also the would-be founders in this space. So buckle up as we go from exploring AI as an intelligence ecosystem to mapping the opportunities in this space and how you can build in it.
Understanding AI as an intelligence ecosystem
Even though it may seem as such, AI is not a linear assembly line where we feed in raw data and get an output. AI can be better understood as an intelligence ecosystem, where data is like the rain that helps lifeforms like applications flourish to generate more data and so on.
.avif)
Every time you dislike a video on YouTube or reroute your car, an entire ecosystem inside and outside of your device changes. One-click tells cloud computers far away to recalibrate your profile, makes small but weighted adjustments to its recommendation algorithm, updates advertisers on your preferences and so forth until you make it learn again. From this lens, let’s look at the various components of this ecosystem and where we are in the AI evolutionary timeline.
The components of an intelligence ecosystem
- Compute: Compute is the hardware and physical environment where all the magic happens, it is the chips and infrastructure of AI. Compute power is measured primarily by one term: Floating Point Operations per second (FLOPs), which measures how many computations it can handle. The more, the better.
- Foundational model: A foundational model is the DNA of the AI. It encodes how the various circuits (neurons) within your compute infrastructure ingest data and produce outputs. For e.g. GPT-3 is a foundational language model that uses deep learning to produce human-like text.
- Fine-tuning: Like any ecosystem, there will be life forms that evolve to specialize and excel in one environment. These retain the core DNA that is the foundational model but improve on them for very specific applications. For e.g. Jasper is a tool that fine-tunes GPT-3 to write specialized marketing copy.
- Applications: Finally we have the end-point applications which is the expression of the data, compute, and foundational model coming together. These are dynamic and interactive. Their sole purpose is to create, learn, and repeat.
You must have seen what these AI intelligence ecosystems can create already but here’s another example of AI describing itself (in poetic verse) as an ecosystem:
AI is an ecosystem of its own
Where algorithms and data interweave
To learn and adapt and grow
A complex network of lines of code
A symphony of machine learning
A tapestry of patterns and insights
A never-ending evolution of intelligence.
- By ChatGPT
This poem, GPT-3, DALL-E, and so many products of the Cambrian explosion of AI applications are examples of AI’s ascension to a new evolutionary stage that we call: Generative AI.
A brief history of AI evolution
Before generative AI there were “rule-based systems”, that involved the development of algorithms to make decisions based on a set of predetermined rules. This type of primitive AI was useful for solving specific problems, but it was limited in its ability to adapt and learn from new data. The fuzzy logic used in the computer inside your washing machine to determine how long it will take to wash your clothes based on multiple inputs is an example of this. AI followed the rules we set without understanding them.
The next stage of AI was machine learning, which involved the development of algorithms that could learn from data and improve their performance over time. This allowed AI systems to become more flexible and adaptable, and to tackle more complex tasks. AI was characterised as “Discriminative” in this phase, primarily used for classification and prediction. TikTok’s algorithm that populates the ‘for you’ page is an example of this. AI not only followed the rules but got better at following them with time.
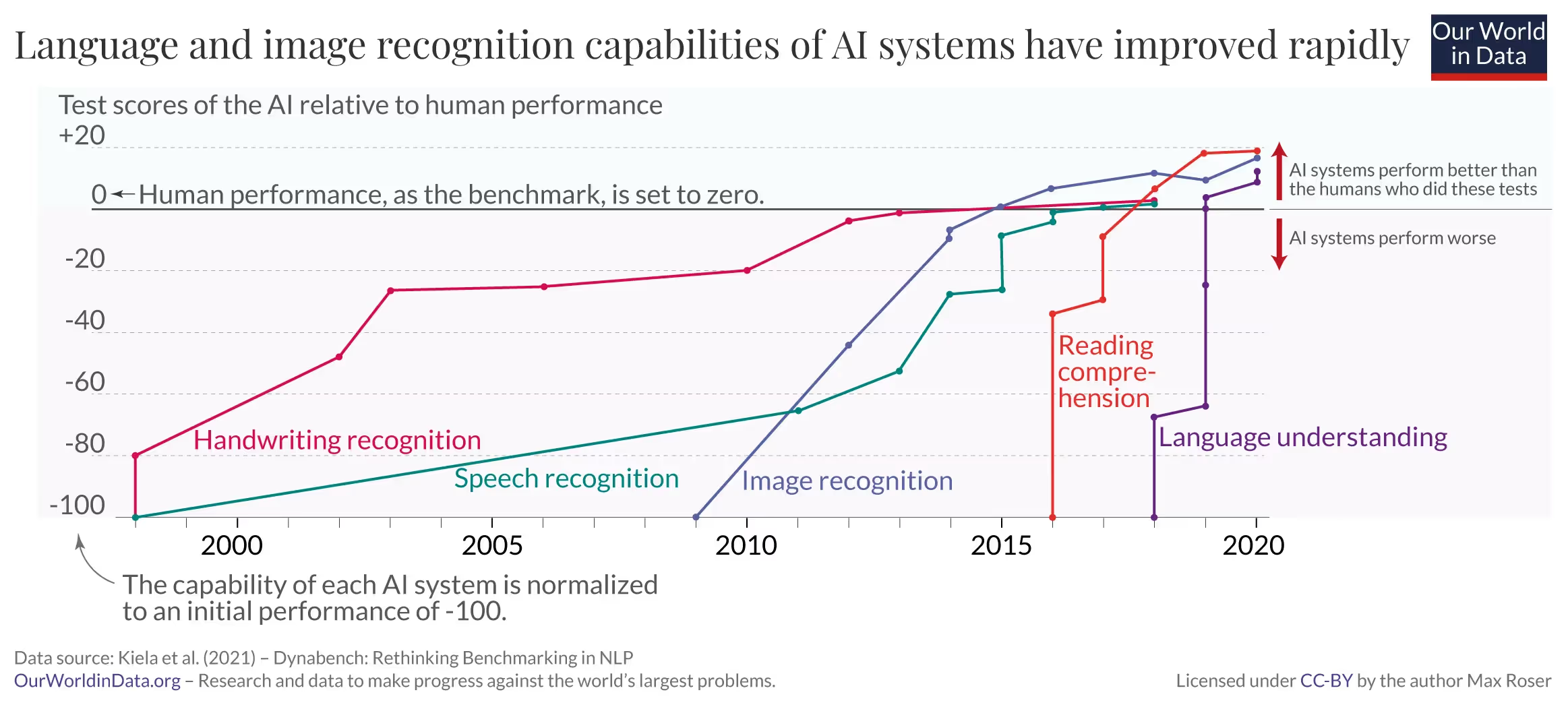
The most recent stage of AI is deep learning, which involves the use of neural networks to learn, ‘understand’ and make decisions. Deep learning models are powerful because they give models a fundamental understanding of concepts and context by training on large amounts of unlabelled data through unsupervised learning. This means the model is not just memorizing what a dog looks like, but developing an understanding of all the components that make up a dog such as tails, whiskers, and ears. These algorithms are not only able to learn, and make predictions and decisions but also create something original. This is the generative phase of AI evolution, where AI could not only understand the rules but play and create with us.
In summary, AI’s evolution has been: Following our rules → Learning our rules → Creating with us → ???
Where AI goes from here is anyone’s guess. An obvious one would be the “redefinition of rules” but before we try to understand what that means let’s look at why this is happening now.
Why now?
Three forces: technological, scientific, and economic have come together to create a perfect storm of innovation in this space.
1. Technological
a) FLOPs beat Moore’s Law
In the last 2 decades or so the number of FLOPs in an AI system has taken off, effectively leaving Moore’s Law in the dust. This has led to AI models making the leap from discriminative to generative by enabling foundational models to train on terabytes of data. From 2016 to today there has been a 100-1000x jump in computing power and it’s doubling every 10 months. As a result, just over the last 3-4 years, models have grown by 15000x in terms of parameters (the number of factors the AI considers while computing). Megatron-Turing Natural Language Generation model is currently the largest transformer model trained on 530 billion parameters!
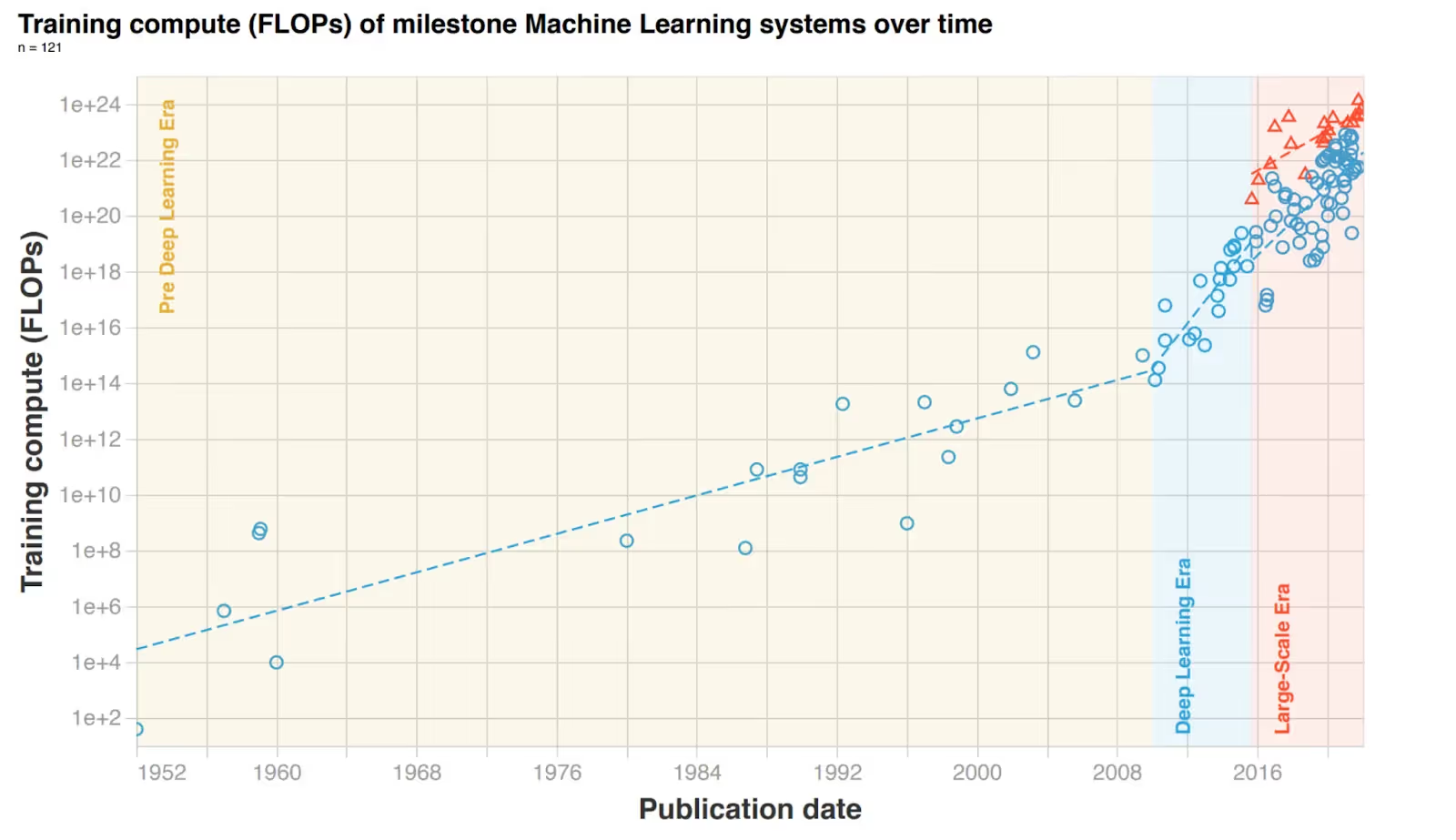
b) NLP and self-driving cars
Two open-ended problems have driven much of the AI development over the last decade or so and have resulted in us reaching this tipping point in AI technology.
- Our quest to understand language for multiple use-cases such as translations or predicting sentences (think auto-complete feature on your email system).
- Trying to solve the problem of operating self-driving cars resulting in step-shift innovations in the field of Computer Vision. This fundamentally improved algorithms that were much superior in understanding the visual world.
Generative AI of today is putting these two together in creative ways by using NLP to understand text prompts and computer vision to produce visual outputs.
2. Scientific
“Attention is all you need”
In 2017, Google Brain researchers published the seminal paper “Attention is all you need” which introduced a ‘transformer’ based foundational model for AI. Transformer architecture is orders of magnitude more efficient than anything that came before. It enabled AI to parallelly compute large amounts of data and preserve some level of context and hierarchy. AI went from 'which one of these photos is a cat?' (discriminative AI) to 'create an image of a cat surfing waves' (generative AI) with the help of transformers. Later researchers used this method for images to help create generative art from the text. DALL-E, GPT-3, Stable Diffusion and most other generative AI models are based on this transformer architecture.

3. Economic
The decentralisation of investments and talent
With the current wave of AI and billions of dollars pouring into the space, we are seeing cutting-edge AI move from research labs to commercial companies, global research collectives, and other more decentralised centres of development.
Companies open-sourcing their large language models through APIs, making them accessible to build on top of, has decentralized and accelerated the pace of innovation.

Where do we go from here?
So where does this leave us? While it is nearly impossible to give definitive predictions, let’s view the possibilities through the lens of common themes that we have seen from previous technology (r)evolutions.
[1] A co-pilot to our creative selves
The majority of knowledge work today is rooted in text, voice/speech, image, video and code. Everything from creating sales pitches, reading legal contracts, coding, and writing scripts will become automated to a large extent.
- Writers will become editors, coders will become engineering managers and so forth. As people’s productivity multiplies, several tasks will become commoditized by an army of customised AI co-pilots.
- As existing professionals amplify their skills, AI will also widen the aperture of who gets to be a coder, artist, game developer, writer and many more knowledge-based professions. This could be the biggest unlock of humanity’s creative potential since the internet.
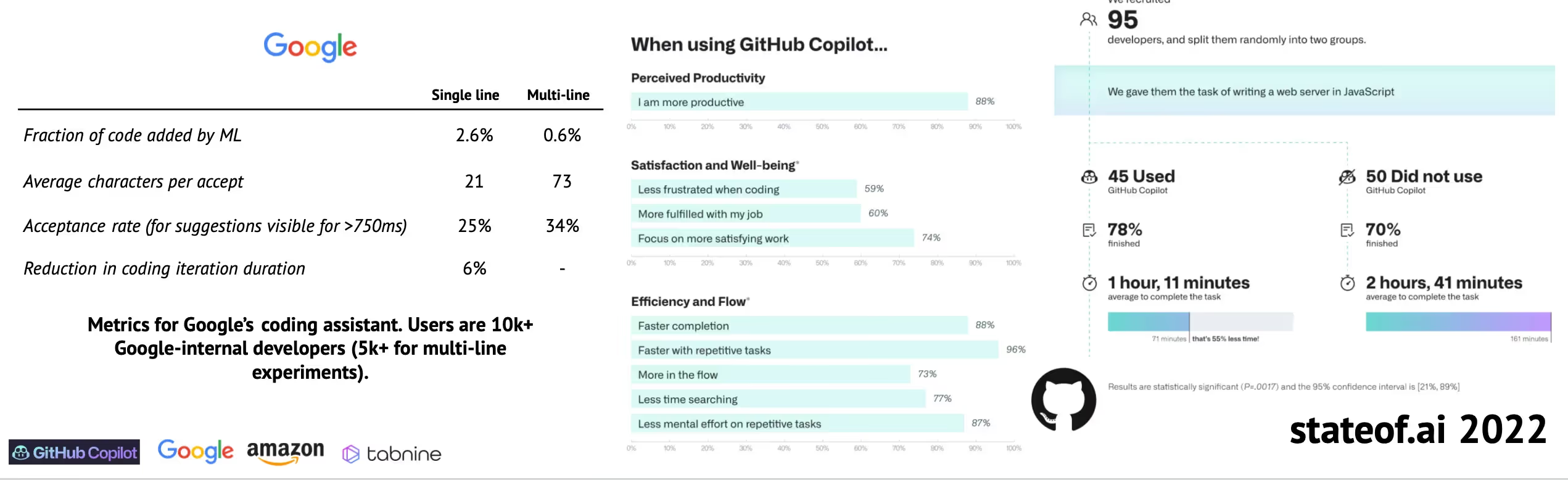
It is important to remember that most jobs won’t go the way of the lamplighter. For high-performance output, human-in-loop workflows will become the norm. In the short and medium term, AI will replace tasks, not jobs.
[2] One prompt → Many outputs
As we go from one input (text) → one output (image or text or code etc.) to one input (text/speech)→ many outputs (text + audio + image + video + code + 3D model etc. ), multi-modal foundational models will be natural successors to DALL-E 2, GPT 3 and other transformer based models of today.
We will eventually transition to a multiplayer experience of using AI that works with each other in different parts of the workflow to help us go from idea to impact faster than ever before. Creators will interweave multiple models that interlock with each other in a modular or lego-like fashion, that are suited to custom workflows.
Imagine thinking of an app and using Codex to code it, Midjourney to create the UI/UX, Meta’s Make A Video to create a promo video and Jasper/Copy.ai to create all the sales and marketing content. We will likely see a more seamless products where different parts of the workflow talk to and learn from each other.
[3] The rise of small custom models that do one thing very well
We are already seeing several custom models emerge on top of large foundational models. This trend will further accelerate.
Startups will continue to 'tune' foundational models to create smaller models for specific verticals. These small models or 'differentiated middleware' will be narrow in scope, but powerful. This could take the form of specialised AI models for medicine, a particular coding language, and more. The Microsoft Research Centre for instance is using fine-tuned AI to help translate and preserve over 800 native Indian languages.
These specialized models will have unique data flywheels that will improve the model over time for that particular vertical, creating enduring value in the process.
Similar to how countries like India directly leapfrogged to mobile phones, a lot of consumers will directly go to using small models at the edge, rather than using large general-purpose models.
[4] A world where bits impact how we manipulate atoms
While technology paradigms like web 3.0, social media, and others usually don’t have a direct impact on the real world of atoms. AI represents a technology that can directly influence how we do science, conduct social studies, and wage war. AI is already amplifying some of the work being done on protein folding, material science, vaccinations, and precision medicine. This is because AI is able to simulate things rapidly with super high fidelity, increasing iteration loops. Some remarkable examples of these are
- Nuclear energy: In Switzerland, DeepMind’s deep RL system is helping scientists in the Swiss Plasma Centre at EPFL control plasma in fusion reactors in a major breakthrough towards achieving sustainable nuclear fusion.
- Defense: In Ukraine, the military is using an AI-powered system called GIS Arta. It ingests data from drones, GPS, forward observers etc. and converts it into dispatch requests for reconnaissance and artillery.
- Medicine: Meta AI’s ESMFold has helped biologists predict the shape of 600 Mn. proteins.
[5] The rise of AI teachers, developers, and guardians
As more AI applications permeate into everyday lives the role of humans in guiding, correcting, and moderating AI will become all the more important. This will be coupled with enterprises integrating and leveraging AI into their workflows and increasing the number of AI/ML developers exponentially.
Similar to how the Asilomar conference on recombinant DNA was pivotal in regulating the proper use of genetic engineering, “AI alignment” and the professions associated with it too will become critical to the fair and ethical use of AI.
[6] Marginal cost of creativity → Zero, Society’s output → ∞
As the marginal cost of creating and intelligence tends to zero, the entire cost structure of society will change like it did when with the internet era. The knowledge value chain will get unbundled creating new points where value is accrued and lost.
From a technology and potential applications PoV, the known unknowns and unknown unknowns for AI are massive. Over a 100 Bn. USD will be invested in this space over the next few years, laying the foundation for future value creation similar to the internet and mobile computing era. The only difference is the "Age of AI" will be bigger and faster.
AI is just getting started
Progress happens sooner and stranger than we think. People believed AI will replace the blue collar jobs, then the low skilled white collar, then high skilled white collar like artists and coders. The above trends, in fact, indicate that AI is moving in the opposite direction.
So, what new companies and movements will AI inspire next? How will they work with each other? And how do you win and capitalise on this once in a decade opportunity as a founder?
These are some of the questions we will explore in the next part of this series as we map and comprehend the fundamental change AI will bring about as a horizontal layer of innovation.
If you are a founder building in the AI space, apply to us at antler.co or write in to isha.dash@antler.co
We mapped the entire Gen-AI landscape across 160 ventures here!